Meet the researcher: Dr. Nanwei Wang, mathematics and statistics
Author: UNB Research
Posted on Jun 1, 2022
Category: Research
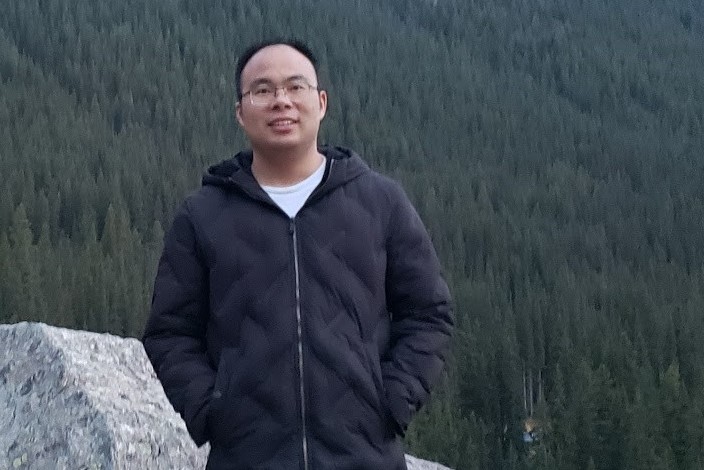
UNB's Dr. Nanwei Wang is using complex mathematics in the fight against cancer. But, how do graphs help fight disease? In this Meet the Researcher story, we speak with Dr. Wang about how math and genetics come together in his research to tackle big, complex challenges.
Who are you?
My name is Dr. Nanwei Wang, and I am a data scientist and assistant professor of mathematics and statistics. I completed my PhD in statistics at York University in 2017, after which I completed a three-year postdoctoral fellowship at Mount Sinai Hospital. I have been at UNB since January 2021, when I was fortunate to get hired here as part of the mathematics and statistics department’s growing data science expertise.
My research is about genomic data analysis with mixed genetic networks. In genome-wide association studies (GWAS), researchers work to determine which genes are strongly correlated to cancer diseases or other genetic diseases. The best, most commonly method in GWAS is linear mixed models, which use regression to try to identify single nucleotide polymorphisms (very common, minute genetic variations in DNA) that have significant correlation with the presence of cancer. However, linear mixed models cannot explore the joint distribution of genetic variables, which is where my research comes in.
What are you currently working on?
In my research, we proposed to use mixed graphical models to integrate different types of genetic variables and to build global genetic networks. My research interests mainly focus on graphical models, Bayesian model selection, linear mixed models and high-dimensional data science.
With graphical models, we use graphs to denote conditional correlations between variables – that is, the relationships between two different factors we are analyzing, based on the other factors present. In this way, we can determine which factors affect each other, and how, in complex sets of data.
A Bayesian model is a statistical model where you use probability to represent all uncertainty within the model – both the uncertainty of the output as well as the uncertainty of the input parameters to the model.
Linear mixed models are an extension of simple linear models to allow both fixed and random effects and are particularly useful when there is interdependence in the data, such as we find in hierarchical structures. Currently, I am working on mixed graphical model learning that integrates genomic data applications.
Why did you decide to work on this research?
I enjoy statistical and data science research work; it is both my career and what I like to do every day! In statistics, mixed graphical models are relatively a new area of theory. Because of this, several theoretical problems need to be proved for mixed graphical models. This is part of what I am working on.
The field of graphical model research is quite active, and there are many great statisticians and data scientists working in it.
With my research, I want to develop new models to help solve complicated, real-world problems, and I would like to see greater application of graphical models in different subjects.
Using graphical or other statistical models to explore the relationships among high dimensional data problems can really help us find the useful information within the large amounts of noise.
One area that I am particularly interested in is computational oncology. My research can be applied to help bioinformatics build genetic networks to study the correlations between genes for different cancer diseases. The project that I am currently working on can provide insight to researchers and help them better understand the genetic effects of cancer disease, and in the future, researchers may be able to develop personalized medical treatments for it.
More information
Dr. Nanwei Wang | Department of Mathematics and Statistics | Faculty of Science
Research at UNB | Graduate Studies at UNB | Postdoctoral fellowships